Can We Read Dataset From External Hard Drive During Training
Abstruse
Artificial intelligence (AI) has shown promise for diagnosing prostate cancer in biopsies. However, results have been limited to individual studies, defective validation in multinational settings. Competitions have been shown to be accelerators for medical imaging innovations, but their bear on is hindered past lack of reproducibility and independent validation. With this in listen, nosotros organized the PANDA claiming—the largest histopathology competition to date, joined by one,290 developers—to catalyze evolution of reproducible AI algorithms for Gleason grading using 10,616 digitized prostate biopsies. We validated that a diverse set of submitted algorithms reached pathologist-level performance on independent cross-continental cohorts, fully blinded to the algorithm developers. On U.s.a. and European external validation sets, the algorithms achieved agreements of 0.862 (quadratically weighted κ, 95% confidence interval (CI), 0.840–0.884) and 0.868 (95% CI, 0.835–0.900) with skilful uropathologists. Successful generalization across different patient populations, laboratories and reference standards, achieved by a variety of algorithmic approaches, warrants evaluating AI-based Gleason grading in prospective clinical trials.
Main
Gleason grading1 of biopsies yields important prognostic information for prostate cancer patients and is a key element for treatment planning2. Pathologists characterize tumors into different Gleason growth patterns based on the histological architecture of the tumor tissue. Based on the distribution of Gleason patterns, biopsy specimens are categorized into ane of five groups, commonly referred to as International Society of Urological Pathology (ISUP) form groups, ISUP form, Gleason grade groups or merely grade groups (GGs)3,4,5,6. This cess is inherently subjective with considerable inter- and intrapathologist variability7,8, leading to both undergrading and overgrading of prostate cancerviii,9,10.
AI algorithms have shown promise for grading prostate cancer11,12, specifically in prostatectomy samples13,14 and biopsiesxv,16,17,18, and by assisting pathologists in the microscopic reviews19,xx. However, AI algorithms are susceptible to various biases in their development and validation21,22. This can result in algorithms that perform poorly outside the cohorts used for their development. Moreover, shortcomings in validating the algorithms' operation on boosted cohorts may lead to such deficiencies in generalization going unnoticed23,24. Algorithms are too frequently developed and validated in a siloed manner: the same researchers who develop the algorithms also validate them. This leads to risks of introducing positive bias, because the developing researchers have command over, for example, establishing the validation cohorts and selecting the pathologists providing the reference standard. At that place has yet to be an independent evaluation of algorithms for prostate cancer diagnosis and grading to assess whether they generalize across different patient populations, pathology labs, digital pathology scanner providers and reference standards derived from intercontinental panels of uropathologists. This represents a cardinal barrier to implementation of algorithms in clinical exercise.
AI competitions have been an effective approach to crowd source the evolution of performant algorithms25,26,27. Despite their effectiveness in facilitating innovation, competitions still tend to endure from a set of limitations. Validation of the resulting algorithms has typically not been performed independently of the algorithm developers. In a competitive setup, the incentive for witting or subconscious introduction of positive bias past the developers is arguably further increased, and a lack of independent validation likewise means that the technical reproducibility of the proposed solutions is not verified. Moreover, competitions have typically not been followed up by validation of the algorithms on additional international cohorts, casting doubt on whether the resulting solutions possess the generalization capability to truly answer the underlying clinical problem, as opposed to being fine-tuned for a particular contest design and dataset28.
Through the present study, we aimed to accelerate the methodology for the design and evaluation of medical imaging AI innovations to develop and rigorously validate the next generation of algorithms for prostate cancer diagnostics. Nosotros organized a global AI competition, the Prostate cANcer form Cess (PANDA) claiming, past compiling and publicly releasing a European (European union) accomplice for AI evolution, the largest publicly available dataset of prostate biopsies to engagement. 2nd, we fully reproduced meridian-performing algorithms and externally validated their generalization to contained Us and EU cohorts and compared them with the reviews of pathologists. The contest setup isolated the developers from the independent evaluation of the algorithms' functioning, minimizing the potential for data leakage and offer a true assessment of the diagnostic power of these techniques. Taken together, nosotros show how the combination of AI and innovative study designs, together with prespecified and rigorous validation across various cohorts, can be utilized to solve challenging and important medical problems.
Results
Characteristics of the datasets
In total, 12,625 whole-slide images (WSIs) of prostate biopsies were retrospectively collected from half dozen dissimilar sites for algorithm evolution, tuning and contained validation (Table 1, Extended Information Fig. 1 and Supplementary Tables 7 and 8). Of these, ten,616 biopsies were available for model development (the development gear up), 393 for performance evaluation during the competition phase (the tuning set), 545 as the internal validation set in the postcompetition phase and 1,071 for external validation.
Cases for development, tuning and internal validation originated from Radboud University Medical Center, Nijmegen, the Netherlands and Karolinska Institutet, Stockholm, Sweden (Extended Data Fig. 1 and Supplementary Methods 1, 2 and 3). The external validation data consisted of a Us and an EU set. The The states set contained 741 cases and was obtained from two contained medical laboratories and a 3rd teaching infirmary. The EU external validation set contained 330 cases and was obtained from the Karolinska University Hospital, Stockholm, Sweden. The histological preparation and scanning of the external validation samples were performed by different laboratories to those responsible for the evolution, tuning and internal validation data.
Reference standards of the datasets
The reference standard for the Dutch function of the training set was determined based on the pathology reports from routine clinical do. For the Swedish part of the training prepare, the reference standard was set by 1 uropathologist (L.Eastward.) post-obit routine clinical workflow. The reference standard for the Dutch part of the internal validation set was adamant through consensus of 3 uropathologists (C.H.v.d.K., R.V. and H.v.B.) from two institutions with 18–28 years of clinical feel afterwards residency (mean of 22 years). For the Swedish subset, four uropathologists (L.E., B.D., H.S. and T.T.) from iv institutions, all with >25 years of clinical feel after residency, gear up the reference standard.
For the US external validation set up, the reference standard was set past a panel of half-dozen US or Canadian uropathologists (Thou.A., A.Due east., T.five.d.K., Yard.Z., R.A. and P.H.) from 6 institutions with 18–34 years of clinical feel afterward residency (mean of 25 years). Each specimen was first reviewed by ii uropathologists from the console. A third uropathologist reviewed discordant cases to arrive at a majority opinion. For this external dataset, immunohistochemistry was bachelor to help in tumor identification. The Eu external validation set up was reviewed by a single uropathologist (L.E.). For details on the uropathologist review protocol, encounter Supplementary Methods 2. On validation sets, the pathologists who contributed to the reference standards showed high pairwise agreement (0.926 on a subset of the EU internal validation set and 0.907 on the US external validation set, Supplementary Table 6). To ensure consistency across dissimilar reference standards, we additionally investigated the understanding between reference standards across continents (Supplementary Table 9). We found high agreement between pathologists across the regions when Eu uropathologists reviewed US data and vice versa. Moreover, bulk votes of the panels were highly consistent with the reference standard of the other region (quadratically weighted κ 0.939 and 0.943 for, respectively, the EU and U.s.a. pathologists, Supplementary Table nine).
Overview of the competition
The written report design of the PANDA challenge was preregistered29 and consisted of a competition and a validation phase. The contest was open up to participants from 21 Apr until 23 July 2020 and was hosted on the Kaggle platform (Supplementary Methods 5). During the contest stage, 1,010 teams, consisting of ane,290 developers from 65 countries, participated and submitted at to the lowest degree one algorithm (Fig. ane). Throughout the competition, teams could request evaluations of their algorithm on the tuning set (Supplementary Methods 2). The algorithms were and so simultaneously blindly validated on the internal validation set (Fig. ii). All teams combined submitted 34,262 versions of their algorithms, resulting in a total of 32,137,756 predictions made past the algorithms.
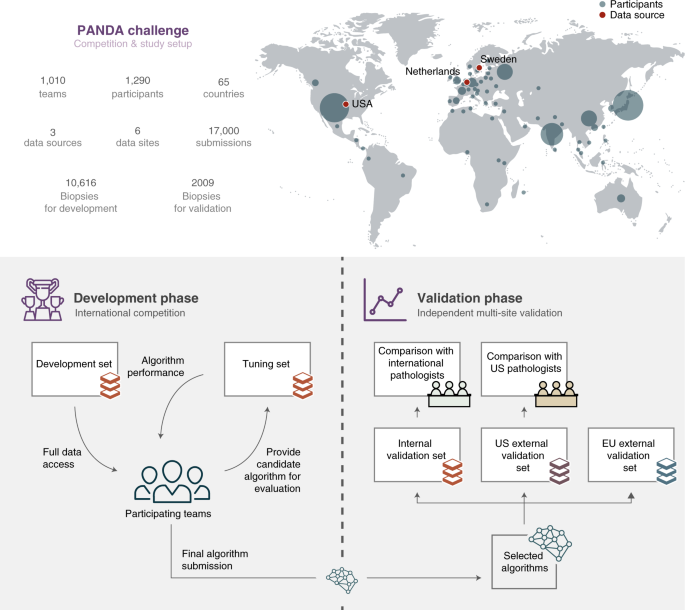
The global competition attracted participants from 65 countries (top: size of the circle for each country illustrates the number of participants). The written report was dissever into ii phases. First, in the development phase (bottom left), teams competed in building the best-performing Gleason grading algorithm, having full access to a development set for algorithm grooming and limited access to a tuning set for estimating algorithm performance. In the validation phase (bottom correct), a selection of algorithms was independently evaluated on internal and external datasets confronting reference grading obtained through consensus across expert uropathologist panels, and compared with groups of international and US general pathologists on subsets of the information.
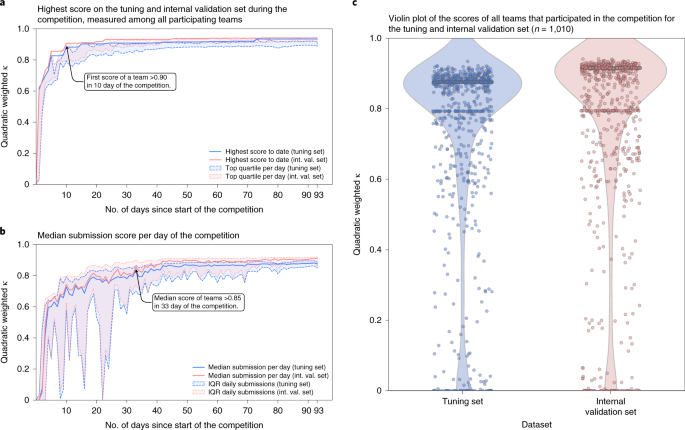
During the competition, teams could submit their algorithm for evaluation on the tuning set, after which they received their score. At the aforementioned time, algorithms were evaluated on the internal validation set, without disclosing these results to the participating teams. a,b, The evolution of the top score obtained by any squad (a) and the median score over all daily submissions (b) throughout the timeline of the competition showing the rapid improvement of the algorithms. c, A big fraction of teams reached loftier scores in the range 0.80–0.90, and retained their performance on the internal validation prepare.
Source information
The first squad to achieve an understanding with the uropathologists of >0.90 (quadratically weighted Cohen's κ) on the internal validation set already occurred within the starting time 10 days of the competition (Fig. ii). In the 33rd mean solar day of the competition, the median performance of all teams exceeded a score of 0.85.
Overview of evaluated algorithms
After the competition, teams were invited to join the PANDA consortium. Of all teams, 33 submitted a proposal to join the validation phase of the report. From these, the competition organizers selected 15 teams based on their algorithm's performance on the internal validation set and method description (Supplementary Methods 6). Among the 10 highest ranking teams in the competition, eight submitted a proposal and were accustomed to join the consortium. A further seven teams in the consortium all ranked inside the competition's meridian xxx.
All selected algorithms made use of deep learning-based methods30,31. Many of the solutions demonstrated the feasibility of terminate-to-end training using case-level information only32, that is, using the International Guild of Urological Pathology (ISUP) GG of a specimen as the target label for an unabridged WSI. Most leading teams, including the winner of the competition, adopted an approach in which a sample of smaller images, or patches, is first extracted from the WSI. The patches are and so fed to a convolutional neural network, the resulting feature responses are concatenated and the final classification layers of the network are practical to these features. This allows preparation a single model terminate-to-end in a computationally efficient fashion to straight predict the ISUP GG of a WSI. Such weakly supervised approaches practice not crave detailed pixel-level annotations as often used in fully supervised training.
Another algorithmic feature adopted past several top-performing teams was to utilise automated characterization cleaning, where samples considered as erroneously graded by the pathologists were either excluded from preparation or relabeled. Several teams indicated the label noise associated with the subjective grading assigned by pathologists as a fundamental problem, and tackled it by algorithms that detect samples where the reference standard deviates considerably from the predictions of the model. Characterization denoising was then typically applied iteratively to refine the labels more aggressively as the model's performance improved during training.
A 3rd key feature shared by all teams of the PANDA consortium was the use of ensembles consisting of various models, featuring, for example, different data preprocessing approaches or unlike neural network architectures. Despite the relative multifariousness in these algorithmic details, past averaging the predictions of the models constituting the ensembles, nearly teams achieved comparable overall performance.
For a summary and details on the individual algorithms see Supplementary Methods vii and Supplementary algorithm descriptions. Most of the evaluated algorithms are available freely for research utilize (please meet Supplementary algorithm descriptions for further details).
Classification performance in the internal validation dataset
In the validation phase, all selected algorithms were fully reproduced on two dissever computing platforms. The boilerplate agreement of the selected algorithms with the uropathologists was high with a quadratically weighted κ of 0.931 (95% CI, 0.918–0.944, Fig. 3). Algorithms showed high sensitivity for tumor detection, with the representative algorithm (selected based on median counterbalanced accuracy, run across Statistical assay) achieving a sensitivity of 99.seven% (95% CI of all algorithms, 98.1–99.7, Fig. 4) and a specificity of 92.9% (95% CI of all algorithms, 91.9–96.7). The nomenclature performances of the private algorithms are presented in Extended Information Figs. ii–4 and Supplementary Tables 2 and 3.

Algorithms' agreement (quadratically weighted κ) with reference standards established by uropathologists shown for the internal and external validation sets (left). On subsets of the internal and U.s. external validation sets, agreement of general pathologists with the reference standards is additionally shown for comparison (right).
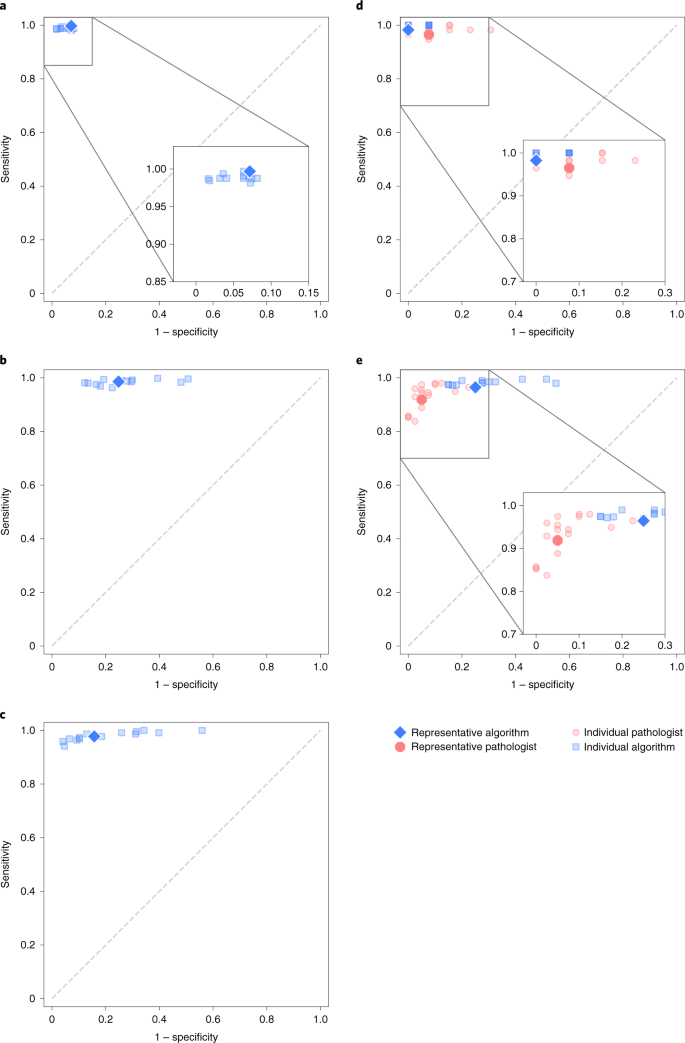
a,b,c, The sensitivity and specificity of the algorithms relative to reference standards established by uropathologists shown for the internal (a) and external validation sets (b, c). b, United states external validation. c, European union external validation. d,e, On subsets of the internal and US external validation sets, the sensitivity and specificity of general pathologists are also shown for comparing. d, International pathologists' comparison. e, US pathologists' comparison.
Nomenclature performance in the external validation datasets
The algorithms were independently evaluated on the two external validation sets. The agreements with the reference standards were high with a quadratically weighted κ of 0.862 (95% CI, 0.840–0.884) and 0.868 (95% CI, 0.835–0.900) for the US and EU external validation sets, respectively. The main algorithm error mode was overdiagnosing of benign cases as ISUP GG i cancer (Extended Data Figs. five and six).
The representative algorithm identified cases with tumor in the external validation sets, with sensitivities of 98.6% (95% CI of all algorithms, 97.6–99.3) and 97.vii% (95% CI of all algorithms, 96.2–99.2) for the US and EU sets, respectively. In comparison to the internal validation fix, the algorithms misclassified more beneficial cases as malignant, resulting in specificities of 75.2% (95% CI of all algorithms, 66.eight–80.0) and 84.3% (95% CI of all algorithms, seventy.5–87.nine) for the representative algorithm.
Classification performance compared with pathologists
To compare algorithms' performances with those of general pathologists, we obtained reviews from 2 panels of pathologists on subsets of the internal and U.s.a. external validation sets. For the Dutch part of the internal validation set, 13 pathologists from 8 countries (7 from Europe and six outside of Europe) reviewed lxx cases. For the US external validation set, twenty US lath-certified pathologists reviewed 237 cases. For details on the pathologist review protocol, see Supplementary Methods 3.
The algorithms scored significantly (P < 0.001) higher in understanding with the uropathologists (0.876, 95% CI, 0.797–0.927; Fig. iii) than the international general pathologists did (0.765, 95% CI, 0.645–0.852) on the seventy cases from the Dutch part of the internal validations set. The representative algorithm had college sensitivity for tumor (98.two%, 95% CI of all algorithms 97.4–100.0) than the representative pathologist (96.5%, 95% CI of all pathologists 95.4–100.0) and higher specificity (100.0%, 95% CI of all algorithms 90.6–100.0, versus 92.iii%, 95% CI of all pathologists 77.8–97.8). On average, the algorithms missed i.0% of cancers, whereas the pathologists missed 1.8%. Differences in grade assignments between the algorithms and pathologists are visualized in Fig. 5.
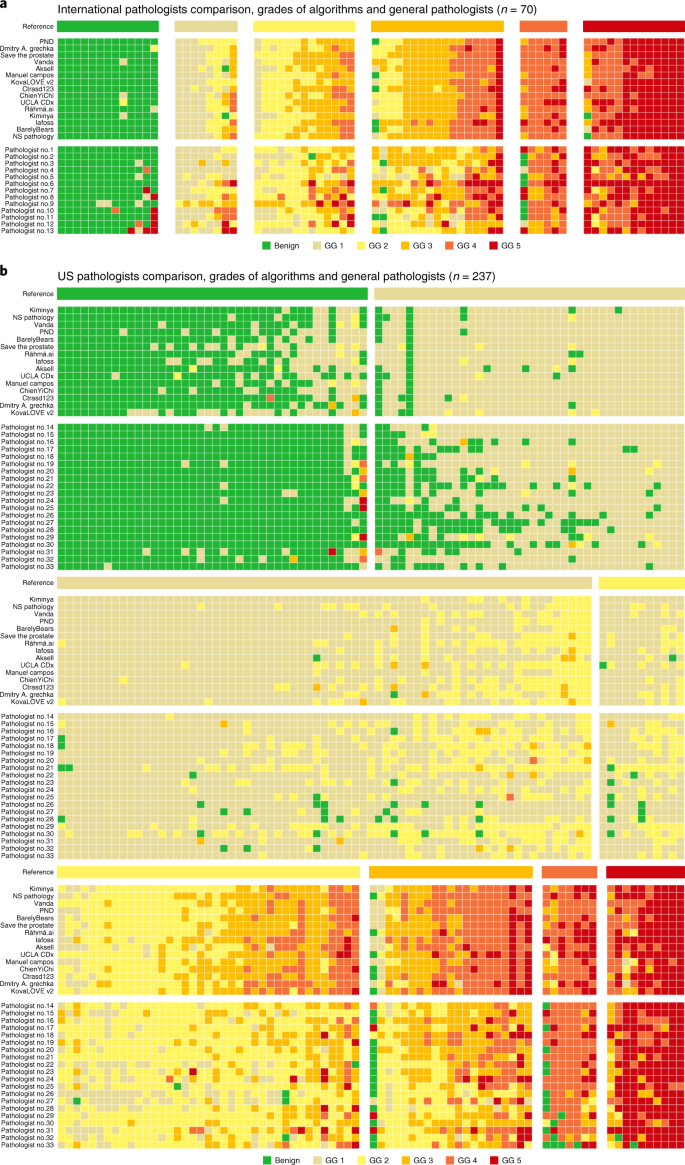
a,b, Algorithms compared with international general pathologists on a subset of the internal validation set (a) and United states general pathologists on a subset of the US external validation set (b). Cases are ordered primarily by the reference ISUP GG and secondarily by the average GG of the algorithms and pathologists. Algorithms and pathologists are ordered past their agreement (quadratically weighted κ) with the reference standard on the corresponding sets. The comparison between pathologists and algorithms gives insight into the deviation in their operating points and for which GGs almost miscalls are made. The algorithms are less likely to miss a biopsy containing cancer, but at the same time more likely to overgrade benign cases.
On the subset of the US external validation set with pathologist reviews, the algorithms exhibited a similar level of understanding with the uropathologists as the US general pathologists did (0.828, 95% CI, 0.781–0.869 versus 0.820, 95% CI, 0.760–0.865; P = 0.53). The representative algorithm had higher sensitivity for tumor (96.four%, 95% CI of all algorithms, 96.6–99.5) than the representative pathologist (91.9%, 95% CI of all pathologists, 89.iii–95.five) but lower specificity (75.0%, 95% CI of all algorithms, 61.2–82.7 versus 95.0%, 95% CI of all pathologists, 87.4–98.1). On average, the algorithms missed ane.nine% of cancers, whereas the pathologists missed seven.3%.
Discussion
AI has shown hope for diagnosis and grading of prostate cancer, merely these results take been restricted to siloed studies with limited proof for generalization across diverse multinational cohorts, representing one of the central barriers to implementation of AI algorithms in clinical practice. The objective of the nowadays study was to overcome these critical issues. First, nosotros aimed to facilitate community-driven development of AI algorithms for cancer detection and grading on prostate biopsies. Second, we sought to transcend isolated assessment of the diagnostic performance of individual AI solutions past focusing on reproducibility and fully blinded validation of a diverse grouping of algorithms on intercontinental and multinational cohorts.
The resulting PANDA claiming was, to the best of our knowledge, the largest competition in pathology organized to date, in terms of both the number of participants and the size of the datasets, and the get-go study to analyze a diversity of AI algorithms for computational pathology on this scale33. The datasets included variability in biopsy sampling procedure, specimen grooming procedure and whole-slide scanning equipment, and had dissimilar and multinational sets of pathologists contributing to the reference standard of the validation sets. Our principal finding was that AI algorithms obtained from a competition setup could successfully detect and grade tumors, reaching pathologist-level cyclopedia with adept reference standards. We farther compared the algorithms with previously published results (Supplementary Table five and Extended Data Fig. seven)15,16,17. The algorithms outperformed earlier works on subsets of the EU validation sets. On the U.s.a. external validation set, the algorithms reached similar performance without any fine-tuning, demonstrating a successful generalization to an unseen independent validation set and beyond any current country of the fine art. Last, groups of international and U.s.a. pathologists also reviewed subsets of the internal and external validation datasets. The algorithms had a concordance with the reference standard that was similar to or college than that of these pathologists.
In the external validation sets, the master algorithm error way was overdiagnosing benign cases every bit ISUP GG 1. This is probably due to the data distribution shift between preparation information and external validation data34, in combination with the study pattern of independent validation, where the teams did non have any admission to the validation sets, potentially leading to suboptimal selection of operating thresholds based only on the tuning fix. We observed this in the The states external validation fix (Fig. 4), where the algorithms appear to exist shifted toward college sensitivity but lower specificity compared with the general pathologists. A potential solution to address the natural information distribution shift is to calibrate the models' predictions using sampled information from the target sites. In addition, we showed high consistency between reference standards (Supplementary Table 9), adding additional proof that the operation drop was not caused past a difference in grading characteristics.
In the U.s. external validation set, tumor identification was confirmed past immunohistochemistry, supporting the finding that the algorithms missed fewer cancers than the pathologists. This college sensitivity shows promise for reducing pathologist workload by automated identification and exclusion of most benign biopsies from review. Analysis of an ensemble synthetic from the algorithms suggests that combining existing algorithms could improve specificity (Supplementary Tabular array four).
Further analysis of the form assignments by the algorithms and general pathologists showed that the algorithms tended to assign higher grades than the pathologists (Fig. 5). For example, in the United states external validation set, algorithms overgraded a substantial portion of ISUP GG iii cases as GG 4. The general pathologists, in contrast, tended to undergrade cases, most notably in the high-course cases. These differences suggest that general pathologists supported by AI could attain higher agreements with uropathologists, potentially alleviating some of the rater variability associated with Gleason grading19,20. Information technology should be noted that the algorithms' operating points were selected solely based on the EU tuning set up. For clinical usage, the operating points tin can be adapted based on the needs and the intended apply cases. For example, for a prescreening utilize case aimed to reduce pathologist workload, one could select an operating indicate favoring high sensitivity to minimize false negatives. Alternatively, if AI was used as a stand-solitary tool, increasing the algorithms' specificity to tumors, while retaining a high sensitivity, could be an of import prerequisite for clinical implementation to foreclose overdiagnosis.
We aimed to lower the entry barrier to medical AI development past providing access to a large, curated dataset, typically attainable only through big enquiry consortia, and past organizing this competition to facilitate articulation development with experience sharing amongst the teams. The results show that the publication of such datasets tin atomic number 82 to rapid development of high-performing AI algorithms. Dissemination and fast iteration of new ideas resulted in the kickoff team achieving pathologist-level performance in the first 10 days of the claiming (Fig. 2). These results show the of import role data play in the evolution of medical AI algorithms, given the brusk pb-time of top-performing solutions by various teams. At the same time, ofttimes raised criticisms of medical AI challenges are the lack of detailed reporting, and express interpretation and reproducibility of results28. Typically algorithms are evaluated only on internal competition data and by participants themselves, which introduces a chance of overfitting and reduced likelihood of reproducibility. Nosotros addressed these limitations in our challenge design by using preregistration, blinded evaluation, full reproduction of algorithm results, contained validation of algorithms on external data and comparison with pathologists.
This study has limitations. Offset, for the validation phase we were express to including 15 teams from the pool of one,010. To ensure transparent selection of teams and minimizing potential bias in the external validation, nosotros disclosed the selection criteria and process beforehand to all participating teams, included both score and algorithm descriptions equally criteria, and performed the choice before running the analyses (Supplementary Methods half-dozen).
Second, algorithm validation was restricted to the assessment of individual biopsies whereas, in clinical practice, pathologists examine multiple biopsies per patient. Future studies can focus on patient-level evaluation of tissue samples, taking multiple cores and sections into business relationship for the last diagnosis. Tertiary, this study focused on grading acinar adenocarcinoma of the prostate, and algorithm responses to other variants and subtypes of cancer, precancerous lesions or nonprostatic tissue were not specifically assessed. Although cases with potential pitfalls were not excluded, it is of involvement to farther examine algorithm functioning on such cases (for case, benign mimickers, severe inflammation, loftier-grade prostatic intraepithelial neoplasia, partial cloudburst) and to investigate which patterns consistently result in classification errors. Although not quantitatively assessed, an analysis of cases with frequent miscalls showed that these cases oftentimes independent patterns such as cutting artifacts, and unlike inflammatory and other biological processes—all common occurrences inside pathology—that could accept resulted in the algorithms' miscalls. A comprehensive understanding of potential fault modes is especially of import when these algorithms go out controlled research settings and are used in clinical settings. Therefore, future inquiry should more extensively appraise what common tissue patterns in pathology routinely affect algorithm performance, whether they are the same patterns that are notoriously hard for pathologists and how we tin can build safeguards to prevent such errors.
Fourth, algorithms were compared against reference standards set past diverse panels of pathologists. Although the gold standard in the field, relying on pathologists' gradings introduces a risk of bias because algorithms could learn the grading habits of specific pathologists and non generalize well to other populations. To remedy this effect, panels of uropathologists established the reference standards of the European union internal and US external validation sets. Although these sets were graded in silo by dissimilar panels, we have shown that a majority-vote reference standard is highly consistent, even in a cross-continental setting (Supplementary Table 9). The EU external validation set was an exception, because a single uropathologist established the reference standard for that prepare. However, nosotros observed loftier concordance between the grading by this pathologist and other pathologists when evaluated on the internal validation set (Supplementary Methods 2). For the training sets, we relied on reference standards extracted from clinical diagnostics, typically set by a single pathologist. Although unfeasible due to the high number of cases, a training reference standard based on multiple pathologists' reviews could take potentially further increased algorithm functioning.
5th, all the information were collected retrospectively across the institutions and the general pathologist reviews were conducted in a nonclinical setting, without additional clinical data bachelor at the time of review. Sixth, despite the international nature of our evaluation (in terms of both pathologists' practise and data sources), the countries involved were predominantly white, and demographic characteristics were not bachelor for all datasets in the present study. Further investigation is required to validate the use of AI algorithms in more various settings35,36. Last, this study did not evaluate the algorithm grading's clan directly with radical prostatectomy or clinical outcomes.
We constitute that a group of AI Gleason grading algorithms developed during a global competition generalized well to intercontinental and multinational cohorts with pathologist-level performance. On all external validation sets, the algorithms achieved high agreement with uropathologists and high sensitivity for malignant biopsies. The performance exhibited by this grouping of algorithms adds evidence of the maturity of AI for this task and warrants evaluation of AI for prostate cancer diagnosis and grading in prospective clinical trials. We foresee a future where pathologists can be assisted by algorithms such as these in the class of a digital colleague. To stimulate further advancement of the field, the total development set of ten,616 biopsies has been made publicly available for noncommercial research apply https://panda.grand-challenge.org/.
Methods
Written report design
The written report design of the PANDA claiming was preregistered29. Nosotros retrospectively obtained and de-identified digitized prostate biopsies with associated diagnosis from pathology reports from Radboud Academy Medical Center, Nijmegen, kingdom of the netherlands and Karolinska Institutet, Stockholm, Sweden (Extended Data Fig. 1 and Supplementary Methods 1, 2 and 3). At the showtime of the competition, participating teams gained admission to this Eu evolution set of 10,616 biopsies from ii,113 patients for training of the AI algorithms (Table i and Supplementary Methods 4). During the course of the competition, the teams could upload their algorithms to the Kaggle platform (Supplementary Methods 5) and receive performance estimates on a tuning set of 393 biopsies. Processing time was limited to six h and the maximum graphics processing unit (GPU) memory bachelor was 16 GB.
By the contest closing date, each squad picked two algorithms of their choice for their last submission, and the higher scoring of the two determined the team'south final ranking. The last evaluation was performed on an internal validation dataset of 545 biopsies, collected from the same sites as the development and tuning sets and fully blinded to the participating teams. Moreover, to obtain an independent internal validation prepare, all samples from a given patient were used for either development or validation.
After the competition on the Kaggle platform ended, all teams were invited to send in a proposal to join the validation stage of the study as members of the PANDA consortium. Joining the validation phase was fully voluntary and not a prerequisite for partaking in the competition. Equally a result, 15 teams were selected for further evaluation on 2 external validation datasets consisting of 741 and 330 biopsies, also fully blinded to the participating teams (Supplementary Methods 6 and seven). The first external validation gear up was obtained from two contained medical laboratories and a tertiary instruction hospital in the Usa. The second external validation set was obtained from the Karolinska University Hospital, Stockholm, Sweden. All datasets consisted of both benign biopsies and biopsies with various ISUP GGs. For details on the inclusion and exclusion criteria, see Supplementary Methods i and Extended Data Fig. 1.
WSIs of the biopsies were obtained using four unlike scanner models from three vendors: 3DHISTECH, Hamamatsu Photonics and Leica Biosystems (Supplementary Table 1). The open up source ASAP software (five.1.9: https://github.com/computationalpathologygroup/ASAP) was used to consign the slides before uploading to the Kaggle platform.
The nowadays study was approved by the institutional review board of Radboud University Medical Heart (IRB 2016-2275), Stockholm regional ideals committee (permits 2012/572-31/1, 2012/438-31/3 and 2018/845-32) and Advarra (Columbia, Doctor; Pro00038251). Informed consent was provided by the participants in the Swedish dataset. For the other datasets, informed consent was waived due to the usage of de-identified prostate specimens in a retrospective setting.
Reproducing algorithms and application to validation sets
All teams selected for the PANDA consortium were asked to provide all information and lawmaking necessary for reproducing the exact version of their algorithm that resulted in the final competition submission. For each algorithm, we collected the master Jupyter notebook or python script for running the inference, the specific Kaggle Docker37 image (https://github.com/Kaggle/docker-python) used by the team during the competition and any necessary associated files, including model weights and auxiliary lawmaking.
We replicated the computational setup of the competition platform and ran the algorithms on two unlike computational systems: Google Cloud and Puhti compute cluster (CSC—IT Middle for Scientific discipline, Espoo, Republic of finland). On the Google Deject platform, all algorithms were run using the original Docker images. On Puhti, the Docker images were automatically converted for use with Singularity38 (v.3.eight.3). The algorithms and scripts provided by the teams were not modified except for minor adjustments required for successful run-fourth dimension installation of dependencies on our computational systems. On Puhti, the algorithms had access to 8 central processing unit (CPU) cores, 32 GB of memory, 1 Tesla V100 32GB GPU (Nvidia) and 500 GB of SSD storage. On the Google Cloud platform, the algorithms had access to 8 CPU cores, 30 GB of memory, 1 Tesla V100 GPU 32GB and 10,000 GB of hard disk drive storage.
Before applying the algorithms on the external validation sets, we first validated that the Kaggle computational environment had been correctly replicated and the algorithms' performance on our systems remained identical. To this end, nosotros ran all algorithms on the tuning and internal validation sets on the two systems to reproduce the output generated during the competition on the Kaggle platform. Past crosschecking the new results with the competition leaderboard, we additionally assured that the algorithms supplied by the teams were not altered later the competition or tuned to perform better on the external validation sets. The verification runs we performed on the Puhti cluster were used as the ground for all results reported on the internal validation set.
Some algorithms were nondeterministic, for example, because of test time augmentations with nonfrozen random seeds. Nosotros ran each of these algorithms five times and averaged the computed metrics.
After verification, nosotros ran all algorithms on the external validation sets. For the U.s.a. external validation set, nosotros used the Google Cloud platform. For the EU external validation ready, we used the Puhti cluster. This process was done independently of the teams and no prior information about the external datasets was supplied to the teams. The ISUP GG predictions of the algorithms on the cases were saved and used equally input for the analysis.
Statistical analysis
We defined the main metric every bit the agreement on ISUP GG with the reference standard of each particular validation set, measured using quadratic Cohen's κ. To compare the performance of the algorithms with that of the general pathologists, we performed a two-sided permutation test per pathologist panel. The average agreement was calculated as the mean of the κ values across the algorithms and the pathologists, respectively. The test statistic was defined every bit the divergence between the average algorithm agreement and the average pathologist agreement.
We calculated sensitivity and specificity on benign versus cancer-containing biopsies for all algorithms and individual general pathologists, based on the reference standard set by the uropathologists. To farther understand how a representative pathologist and algorithm performed, we selected the pathologist and the algorithm with the median balanced accurateness (the average of sensitivity and specificity) as the representative pathologist and the representative algorithm, and reported the associated sensitivity and specificity. A representative pathologist or algorithm was used in favor of averaging across algorithms and pathologists for better estimates of performance. For the 95% CIs of the algorithms' and pathologists' performance metrics, we used bootstrapping beyond all algorithms or pathologists, with both the algorithm or pathologist and case equally the resampling unit of measurement.
Assay was performed using scripts39 written in Python (v.3.eight) in combination with the following software packages: scipy (1.5.4), pandas (1.1.four), mlxtend (0.18.0), numpy (ane.xix.4), scikit-larn (0.23.2), matplotlib (3.3.2), jupyterlab (2.2.ix) and notebook (vi.one.five).
Reporting Summary
Further information on research design is available in the Nature Enquiry Reporting Summary linked to this commodity.
Data availability
The total evolution set up, from hither on named the PANDA challenge dataset, of 10,616 digitized de-identified hematoxylin and eosin-stained prostate biopsies, will be fabricated publicly available for further research. The data can be used nether a Artistic Eatables BY-SA-NC 4.0 license. To attach to the 'Attribution' office of the license, we ask anyone who uses the data to cite the current commodity. The well-nigh upwards-to-date information regarding the dataset is available at the claiming website at https://panda.grand-challenge.org. Source information are provided with this paper.
Code availability
Code that was used to generate the results of the diverse algorithms, and example lawmaking on how to load the images in the PANDA dataset is available at https://github.com/DIAGNijmegen/panda-challenge and https://doi.org/10.5281/zenodo.5592578. Algorithms were congenital using open up source deep learning frameworks, including Pytorch (https://pytorch.org) and TensorFlow (https://world wide web.tensorflow.org). The Docker prototype that all the algorithms were based on is available online at https://github.com/Kaggle/docker-python. Details on the availability of specific models and the code of the contributed algorithms can be constitute in the Supplementary algorithm descriptions.
References
-
Epstein, J. I. An update of the gleason grading system. J. Urol. 183, 433–440 (2010).
-
Mohler, J. L. et al. Prostate cancer, version 2.2019, NCCN clinical do guidelines in oncology. J. Natl Compr. Canc. Netw. 17, 479–505 (2019).
-
van Leenders, G. J. L. H. et al. The 2019 international society of urological pathology (ISUP) consensus briefing on grading of prostatic carcinoma. Am. J. Surg. Pathol. 44, e87 (2020).
-
Epstein, J. I. et al. The 2014 International Society of Urological Pathology (ISUP) consensus conference on gleason grading of prostatic carcinoma: Definition of grading patterns and proposal for a new grading system. Am. J. Surg. Pathol. 40, 244–252 (2016).
-
Pierorazio, P. Grand., Walsh, P. C., Partin, A. W. & Epstein, J. I. Prognostic Gleason grade grouping: data based on the modified Gleason scoring system. BJU Int. 111, 753–760 (2013).
-
Epstein, J. I. et al. A contemporary prostate cancer grading organisation: a validated culling to the gleason score. Eur. Urol. 69, 428–435 (2016).
-
Allsbrook, W. C. Jr et al. Interobserver reproducibility of Gleason grading of prostatic carcinoma: urologic pathologists. Hum. Pathol. 32, 74–fourscore (2001).
-
Melia, J. et al. A United kingdom-based investigation of inter- and intra-observer reproducibility of Gleason grading of prostatic biopsies. Histopathology 48, 644–654 (2006).
-
Ozkan, T. A. et al. Interobserver variability in Gleason histological grading of prostate cancer. Scand. J. Urol. 50, 420–424 (2016).
-
Egevad, L. et al. Standardization of Gleason grading amidst 337 European pathologists. Histopathology 62, 247–256 (2013).
-
Goldenberg, S. L., Nir, Thousand. & Salcudean, S. E. A new era: bogus intelligence and machine learning in prostate cancer. Nat. Rev. Urol. sixteen, 391–403 (2019).
-
Nir, Grand. et al. Comparison of artificial intelligence techniques to evaluate performance of a classifier for automated grading of prostate cancer from digitized histopathologic images. JAMA Netw. Open 2, e190442 (2019).
-
Han, W. et al. Histologic tissue components provide major cues for machine learning-based prostate cancer detection and grading on prostatectomy specimens. Sci. Rep. 10, 9911 (2020).
-
Nagpal, K. et al. Development and validation of a deep learning algorithm for improving Gleason scoring of prostate cancer. NPJ Digit. Med. ii, 48 (2019).
-
Nagpal, Chiliad. et al. Development and validation of a deep learning algorithm for gleason grading of prostate cancer from biopsy specimens. JAMA Oncol. six, 1372–1380 (2020).
-
Bulten, W. et al. Automatic deep-learning system for Gleason grading of prostate cancer using biopsies: a diagnostic study. Lancet Oncol. 21, 233–241 (2020).
-
Ström, P. et al. Bogus intelligence for diagnosis and grading of prostate cancer in biopsies: a population-based, diagnostic study. Lancet Oncol. 21, 222–232 (2020).
-
Kott, O. et al. Evolution of a deep learning algorithm for the histopathologic diagnosis and gleason grading of prostate cancer biopsies: a pilot written report. Eur. Urol. Focus 7, 347–351 (2021).
-
Steiner, D. F. et al. Evaluation of the utilize of combined artificial intelligence and pathologist cess to review and grade prostate biopsies. JAMA Netw. Open 3, e2023267 (2020).
-
Bulten, West. et al. Artificial intelligence assistance significantly improves Gleason grading of prostate biopsies by pathologists. Mod. Pathol. https://doi.org/10.1038/s41379-020-0640-y (2020).
-
Challen, R. et al. Artificial intelligence, bias and clinical condom. BMJ Qual. Saf. 28, 231–237 (2019).
-
Nagendran, M. et al. Artificial intelligence versus clinicians: systematic review of blueprint, reporting standards, and claims of deep learning studies. BMJ 368, m689 (2020).
-
AI diagnostics need attending. Nature 555, 285–285 (2018).
-
Yasaka, M. & Abe, O. Deep learning and artificial intelligence in radiology: current applications and future directions. PLoS Med. 15, e1002707 (2018).
-
Ehteshami Bejnordi, B. et al. Diagnostic assessment of deep learning algorithms for detection of lymph node metastases in women with breast cancer. JAMA 318, 2199–2210 (2017).
-
Bandi, P. et al. From detection of private metastases to classification of lymph node condition at the patient level: the CAMELYON17 challenge. IEEE Trans. Med. Imaging 38, 550–560 (2019).
-
Caicedo, J. C. et al. Nucleus division across imaging experiments: the 2018 Data Scientific discipline Basin. Nat. Methods 16, 1247–1253 (2019).
-
Maier-Hein, L. et al. Why rankings of biomedical image analysis competitions should exist interpreted with care. Nat. Commun. ix, 5217 (2018).
-
Bulten, West. et al. The PANDA challenge: Prostate cANcer form Assessment using the Gleason grading system. Zenodo https://doi.org/10.5281/zenodo.3715938 (2020).
-
LeCun, Y., Bengio, Y. & Hinton, G. Deep learning. Nature 521, 436–444 (2015).
-
Litjens, G. et al. A survey on deep learning in medical paradigm analysis. Med. Image Anal. 42, sixty–88 (2017).
-
van der Laak, J., Ciompi, F. & Litjens, G. No pixel-level annotations needed. Nat. Biomed. Eng. 3, 855–856 (2019).
-
Hartman, D. J., Van Der Laak, J. A. Due west. One thousand., Gurcan, Chiliad. Northward. & Pantanowitz, L. Value of public challenges for the evolution of pathology deep learning algorithms. J. Pathol. Inform. 11, 7 (2020).
-
Kelly, C. J., Karthikesalingam, A., Suleyman, K., Corrado, One thousand. & King, D. Cardinal challenges for delivering clinical bear on with bogus intelligence. BMC Med. 17, 195 (2019).
-
Rajkomar, A., Hardt, M., Howell, G. D., Corrado, Grand. & Mentum, M. H. Ensuring fairness in machine learning to advance health disinterestedness. Ann. Intern. Med. 169, 866–872 (2018).
-
Gianfrancesco, M. A., Tamang, S., Yazdany, J. & Schmajuk, G. Potential biases in machine learning algorithms using electronic wellness tape information. JAMA Intern. Med. 178, 1544–1547 (2018).
-
Merkel, D. Docker: lightweight Linux containers for consequent development and deployment. Linux J. 2014, ii (2014).
-
Kurtzer, G. M., Sochat, V. & Bauer, M. W. Singularity: scientific containers for mobility of compute. PLoS I 12, e0177459 (2017).
-
Bulten, W. et al. PANDA challenge analysis code. Zenodo https://doi.org/x.5281/zenodo.5592578 (2020).
Acknowledgements
We were supported past the Dutch Cancer Gild (grant no. KUN 2015-7970, to Due west.B., H.P. and Thou.L.); Netherlands Organisation for Scientific Research (grant no. 016.186.152, to G.50.); Google LLC, Verily Life Sciences, Swedish Inquiry Quango (grant nos. 2019-01466 and 2020-00692, to 1000.E.); Swedish Cancer Society (Tin can, grant no. 2018/741, to M.E.); Swedish eScience Research Centre, EIT Wellness, Karolinska Institutet, Åke Wiberg Foundation and Prostatacancerförbundet (all to Grand.E.); Academy of Finland (grant nos. 341967 and 335976, to P.Ruusuvuori), Cancer Foundation Finland (project 'Computational pathology for enhanced cancer grading and patient stratification', to P.Ruusuvuori) and ERAPerMed (grant no. 334782, 2020-22, to P.Ruusuvuori). Google LLC approved the publication of the manuscript, and the remaining funders had no role in written report pattern, data collection and analysis, decision to publish or training of the manuscript. Nosotros thank the MICCAI lath challenge working group, the MICCAI 2020 satellite issue team and the MICCAI claiming reviewers for their support in the challenge workshop and review of the challenge pattern. We give thanks Kaggle for hosting the contest, providing compute resources and the competition prizes. We give thanks CSC—It Center for Scientific discipline, Finland, for providing computational resources. We thank E. Wulczyn, A. Um'rani, Y. Liu and D. Webster for their feedback on the manuscript and guidance of the project. We thank our collaborators at NMCSD, particularly Due north. Olson, for internal reuse of de-identified information, which contributed to the United states of america external validation prepare.
Writer information
Affiliations
Consortia
Contributions
W.B., K.Kartasalo and P.-H.C.C. had full access to all of the data in the written report and take responsibleness for the integrity of the information and the accuracy of the data assay. P.S., H.P. and Yard.Due north. contributed equally to this piece of work. W.B., Chiliad.Kartasalo, P.-H.C.C., P.S., P.Ruusuvuori, M.E. and G.L. conceived the project. W.B., K.Kartasalo, P.-H.C.C., P.S., H.P., Thou.Due north., Y.C., D.F.S., H.five.B., R.V., C.H.-v.d.K., J.v.d.L., H.S., B.D., T.T., T.H., H.Grand., 50.Eastward., P.Ruusuvuori, G.L., M.E., A.B., A.Ç., X.F., M.G., V.M., G.P., P.Roy, Thou.S., P.K.O.S., E.Southward., J.T., J.B.-L., E.K.P., Thousand.B.A., A.J.E., T.v.d.K., M.Z., R.A., P.A.H., M.V. and F.T. curated the data. Westward.B., One thousand.Kartasalo, P.-H.C.C., Chiliad.Northward., P.Ruusuvuori, G.East. and G.L. did the formal analysis. P.-H.C.C., G.S.C., 50.P., C.H.M., G.Fifty., J.v.d.Fifty., P.Ruusuvuori and 1000.E. acquired the funding. Westward.B., Grand.Kartasalo, P.-H.C.C., K.N., P.Ruusuvuori, M.Due east. and G.L. did the investigations. West.B., K.Kartasalo, P.-H.C.C., P.S., K.N., P.Ruusuvuori, M.Eastward. and G.L. provided the methodology. W.B., K.Kartasalo and M.D. administered the projection. W.B., D.F.S., S.D. and P.Ruusuvuori provided the resources. West.B., K.Kartasalo, H.P., P.-H.C.C., K.N., Y.C., South.D., One thousand.V.S., One thousand.Z., J.J., A.R., Y.F., K.Y., W.50., J.Fifty, W.S., C.A., R.K., R.G., C.-50.H., Y.Z., H.S.T.B., V.K., D.V., Five.M., E.50., H.Y., T.Y., M.O., T.Southward., T.C., N.W., N.O., S.Y., Thou.Kim, B.B., Y.West.M., H.-S.50., J.P., M.C., D.G., S.H., S.S., Q.Due south, J.J., Grand.T. and A.K. provided the software. P.-H.C.C., 50.P., C.H.M., P.Ruusuvuori, Thousand.E., Thousand.L. and J.v.d.L. supervised the project. Due west.B., M.Kartasalo, P.-H.C.C., H.P. and K.N. validated the report. Westward.B. visualized the study. Due west.B., Grand.Kartasalo, P.-H.C.C., P.Ruusuvuori, Yard.E. and Thou.50. wrote the original draft of the manuscript. Due west.B., K.Kartasalo, P.-H.C.C., K.Northward., H.P., P.Southward., K.N., Y.C., D.F.S., H.v.B., R.V., C.H.-v.d.K., J.v.d.L., H.G., H.Due south., B.D., T.T., T.H., Fifty.E., M.D., Southward.D., L.P., C.H.Chiliad. P.Ruusuvuori, Grand.Due east., Chiliad.50., A.B., A.Ç., XF., G.G., 5.K., Chiliad.P., P.Roy, G.Southward., P.G.O.S., East,S., J.T., J.B.-L., E.M.P., M.B.A., A.J.East., T.v.d.K., 1000.Z., R.A., P.A.H., Chiliad.V.Due south., J.J., A.R., K.Z., Y.F., K.Y., West.L., JL., Due west.S., C.A., R.K., R.Yard., C.-L.H., I.Z., H.S.T.B., V.K., D.V., V.Chiliad., East.L., H.Y., T.Y., K.O., T.S., T.C. Due north.W., N.O., Southward.Y., Thousand.Kim, B.B., Y.Westward.K., H.-S.L., J.P., M.C., D.G., S.H., Due south.Southward., Q.S., J.J., M.T. and A.K. reviewed and edited the manuscript.
Corresponding authors
Ethics declarations
Competing interests
West.B. and H.P. report grants from the Dutch Cancer Society, during the conduct of the present study. J.v.d.50. reports consulting fees from Philips, ContextVision and AbbVie, and grants from Philips, ContextVision and Sectra, exterior the submitted work. M.L. reports grants from the Dutch Cancer Society and the NWO, during the behave of the present study, and grants from Philips Digital Pathology Solutions and personal fees from Novartis, outside the submitted work. M.E. reports grants from Swedish Research Council, Swedish Cancer Society, Swedish eScience Enquiry Centre, EIT Health, Karolinska Institutet, Åke Wiberg Foundation and Prostatacancerförbundet. P.Ruusuvuori reports grants from Academy of Finland, Cancer Foundation Finland and ERAPerMed. H.Thousand. has five patents (WO2013EP7425920131120, WO2013EP74270 20131120, WO2018EP52473 20180201, WO2015SE50272 20150311 and WO2013SE50554 20130516) related to prostate cancer diagnostics pending, and has patent applications licensed to A3P Biomedical. M.E. has four patents (WO2013EP74259 20131120, WO2013EP74270 20131120, WO2018EP52473 20180201 and WO2013SE50554 20130516) related to prostate cancer diagnostics pending, and has patent applications licensed to A3P Biomedical. P.-H.C.C., G.N., Y.C., D.F.S., M.D., S.D., F.T., K.S.C., L.P. and C.H.One thousand. are employees of Google LLC and own Alphabet stock, and written report several patents granted or pending on machine-learning models for medical images. Grand.B.A. reported receiving personal fees from Google LLC during the conduct of the nowadays written report and receiving personal fees from Precipio Diagnostics, CellMax Life and IBEX outside the submitted piece of work. A.E. is employed by Mackenzie Health, Toronto. T.five.d.K. is employed by University Health Network, Toronto; the time spent on the project was supported by a enquiry agreement with financial support from Google LLC. R.A. and P.A.H. were compensated by Google LLC for their consultation and annotations as expert uropathologists. H.Y. reports nonfinancial support from Aillis Inc. during the conduction of the present study. Westward.L., J.L., W.S. and C.A. have a patent (US 62/852,625) awaiting. 1000.Kim, B.B., Y.Due west. Chiliad., H.-S.L. and J.P. are employees of VUNO Inc. M.B.A. reported receiving personal fees from Google LLC during the conduct of the present study and receiving personal fees from Precipio Diagnostics, CellMax Life and IBEX outside the submitted work. A.Due east. is employed by Mackenzie Health, Torontoa. T.v.d.K. is employed by University Wellness Network, Toronto; the time spent on the project was supported by a research understanding with fiscal support from Google LLC. M.Z., R.A. and P.A.H. were compensated by Google LLC for their consultation and annotations every bit expert uropathologists. All other authors declare no competing interests.
Peer review information
Nature Medicine thanks Moritz Gerstung, Jonathan Epstein, Priti Lal and the other, anonymous, reviewer(s) for their contribution to the peer review of this work. Javier Carmona was the master editor on this article and managed its editorial process and peer review in collaboration with the remainder of the editorial team.
Additional information
Publisher's notation Springer Nature remains neutral with regard to jurisdictional claims in published maps and institutional affiliations.
Extended data
Supplementary information
Source data
Source Information Fig. ii
Progression of algorithms' performances (highest score and median) throughout the competition on the tuning and internal validation set.
Source Data Extended Information Fig. vii
Functioning of individual algorithms compared with prior work on validation (sub)sets of those earlier works.
Rights and permissions
Open Access This article is licensed under a Creative Commons Attribution iv.0 International License, which permits apply, sharing, adaptation, distribution and reproduction in any medium or format, as long as yous give advisable credit to the original writer(s) and the source, provide a link to the Creative Commons license, and signal if changes were made. The images or other 3rd political party fabric in this article are included in the article's Creative Eatables license, unless indicated otherwise in a credit line to the material. If material is not included in the article'south Creative Commons license and your intended use is not permitted by statutory regulation or exceeds the permitted employ, you lot will need to obtain permission directly from the copyright holder. To view a copy of this license, visit http://creativecommons.org/licenses/by/4.0/.
Reprints and Permissions
About this article
Cite this article
Bulten, Due west., Kartasalo, K., Chen, PH.C. et al. Artificial intelligence for diagnosis and Gleason grading of prostate cancer: the PANDA challenge. Nat Med 28, 154–163 (2022). https://doi.org/10.1038/s41591-021-01620-ii
-
Received:
-
Accepted:
-
Published:
-
Issue Date:
-
DOI : https://doi.org/x.1038/s41591-021-01620-2
wilkersoncasim1999.blogspot.com
Source: https://www.nature.com/articles/s41591-021-01620-2
0 Response to "Can We Read Dataset From External Hard Drive During Training"
Publicar un comentario